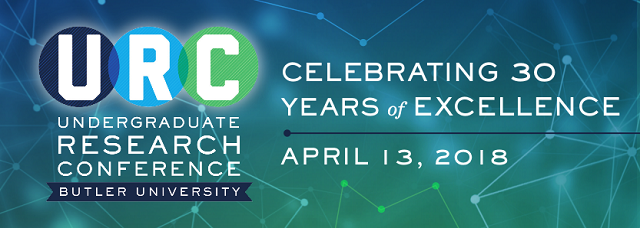
Mathematics & Computer Science
Data Analysis: Estimation of Parameters Using Bayes Theorem
Document Type
Poster Presentation
Location
Indianapolis, IN
Start Date
13-4-2018 8:30 AM
End Date
13-4-2018 10:00 AM
Sponsor
Arbin Thapaliya (Franklin College)
Description
Fitting parameters of interest in an elegant and efficient way via analysis of experimental data is one of the important steps towards obtaining accurate central values for these parameters. The Bayesian method based on the probability theory is one of the simplest and straightforward methods for statistical analysis of data. In our consideration, we generate pseudo-data from a simple function that will serve to demonstrate the applicability of the Bayesian method. The choice of this particular function is made because its power-series expansion generates coefficients that have absolute values all of order 1. This is particularly important because this requirement will enable us to define a prior which is one of the important steps towards applying the Bayesian method. Often, there can be certain parameters that enter into our calculations that we are not interested in evaluating. One other interesting feature of the Bayesian approach is the so called, marginalization, which integrates these unwanted parameters out of the probability density function leaving only the parameters that we want to obtain. We plan to incorporate marginalization in our future calculations. As far as our current analysis is concerned, we explicitly demonstrate how the use of the Bayesian method produces good estimation of parameters of interest. Also, the errors for the estimations using Bayesian analysis turned out to by a factor of 10 or more in our analysis.
Data Analysis: Estimation of Parameters Using Bayes Theorem
Indianapolis, IN
Fitting parameters of interest in an elegant and efficient way via analysis of experimental data is one of the important steps towards obtaining accurate central values for these parameters. The Bayesian method based on the probability theory is one of the simplest and straightforward methods for statistical analysis of data. In our consideration, we generate pseudo-data from a simple function that will serve to demonstrate the applicability of the Bayesian method. The choice of this particular function is made because its power-series expansion generates coefficients that have absolute values all of order 1. This is particularly important because this requirement will enable us to define a prior which is one of the important steps towards applying the Bayesian method. Often, there can be certain parameters that enter into our calculations that we are not interested in evaluating. One other interesting feature of the Bayesian approach is the so called, marginalization, which integrates these unwanted parameters out of the probability density function leaving only the parameters that we want to obtain. We plan to incorporate marginalization in our future calculations. As far as our current analysis is concerned, we explicitly demonstrate how the use of the Bayesian method produces good estimation of parameters of interest. Also, the errors for the estimations using Bayesian analysis turned out to by a factor of 10 or more in our analysis.